ADHD in Artificial Intelligence: Modeling Diverse Minds
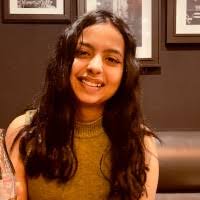

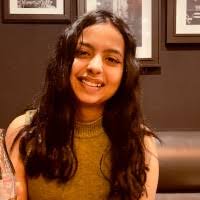
As we venture deeper into the age of artificial intelligence, researchers and developers are increasingly looking to the human mind for inspiration. One fascinating area of exploration is how AI can model and learn from diverse cognitive styles, including those associated with neurodevelopmental conditions like Attention Deficit Hyperactivity Disorder (ADHD). This intersection of AI and ADHD opens up intriguing possibilities for both fields, potentially leading to more comprehensive technology and better understanding of neurodiversity.
ADHD is characterized by symptoms such as inattention, hyperactivity, and impulsivity. These ADHD symptoms can present challenges in traditional settings, but they also come with unique strengths like creativity, hyperfocus, and out-of-the-box thinking. As AI researchers strive to create more human-like intelligence, incorporating these diverse cognitive patterns could lead to more flexible and innovative AI systems.
One way ADHD is influencing AI development is through the concept of "attention mechanisms" in machine learning models. These mechanisms allow AI systems to focus on relevant information while filtering out noise – a process that mimics human attention. By studying how individuals with ADHD manage attention differently, particularly those with ADHD with inattention, researchers can potentially design more adaptable attention mechanisms in AI, leading to systems that can switch focus rapidly or hyperfocus on specific tasks when needed.
Another area where ADHD insights are proving valuable is in reinforcement learning, a type of machine learning where an AI agent learns through trial and error. The impulsivity and risk-taking tendencies often associated with ADHD could inspire AI models that are more exploratory and willing to try unconventional solutions. This could be particularly useful in complex problem-solving scenarios where traditional approaches fall short.
Moreover, the non-linear thinking patterns common in individuals with ADHD could inform the development of AI systems that excel at creative tasks and lateral thinking. By incorporating these cognitive styles, we might see AI that's better at generating novel ideas, making unexpected connections, and approaching problems from unique angles.
It's important to note that modeling ADHD in AI isn't about replicating a condition, but rather about learning from the diverse ways human minds can operate. This approach aligns with the broader movement towards neurodiversity, which recognizes and values the full range of human cognitive variations, including different ADHD types.
As we continue to explore the potential of AI inspired by diverse cognitive styles, we're also seeing advancements in how AI can assist in understanding and assessing for neurodevelopmental conditions. An exciting development in this area is Simon AI, a cutting-edge tool that can objectively identify ADHD signs from just a 60-second voice sample using artificial intelligence. This innovative approach demonstrates how AI can not only learn from diverse minds but also help in their assessment and understanding, providing a personalised neuro profile for all.
The effects of ADHD on cognitive processes can be both challenging and advantageous, and AI researchers are keen to explore how these unique thought patterns can enhance machine learning models.
In conclusion, the intersection of ADHD and AI represents a fascinating frontier in cognitive science and technology. By embracing neurodiversity in AI development, we're not only creating more comprehensive and versatile systems but also gaining deeper insights into the beautiful complexity of the human mind. As tools like Simon AI show us, this synergy between AI and neurodevelopmental research has the potential to revolutionize how we approach cognitive diversity, both in technology and in society at large, potentially leading to better understanding and management of ADHD effects across various domains.